What is semantic search, and how does it enhance enterprise productivity?
.webp)
Semantic search represents a paradigm shift in the way we search for and retrieve information. Unlike conventional keyword searches that rely solely on matching words or phrases, semantic search seeks to understand the context, meaning, and intent behind user queries. By analyzing the relationships between words, concepts, and entities, semantic search engines can deliver more precise and relevant results.
How does semantic search work in enterprise settings?
In enterprise settings, the complexity of data landscapes often presents significant challenges for employees seeking to access relevant information efficiently. Semantic search emerges as a powerful solution tailored to address these challenges head-on. Leveraging sophisticated natural language processing (NLP) techniques and machine learning algorithms, semantic search platforms transform the way employees interact with data.
Here's how semantic search works in enterprise environments:
Understanding user intent: Semantic search goes beyond mere keyword matching by delving into the underlying intent behind user queries. By analyzing the semantics of the language, including context, synonyms, and user preferences, these platforms decipher the true meaning behind each query.
Conceptual mapping: Instead of relying solely on exact keyword matches, semantic search engines employ advanced algorithms to map the relationships between words, concepts, and entities. This enables them to grasp the nuances of user queries and retrieve information that aligns with the user's underlying intent.
Contextual relevance: In enterprise environments, where data is dispersed across diverse repositories and applications, contextual relevance is paramount. Semantic search platforms excel at understanding the context surrounding each piece of information, ensuring that search results are tailored to the specific needs of the user and the task at hand.
Cross-platform integration: One of the key strengths of semantic search in enterprise settings is its ability to seamlessly integrate with a wide range of data sources and applications. Whether it's structured data from databases, unstructured data from documents, or real-time data streams, semantic search platforms can aggregate information from disparate sources to provide a unified search experience.
Continuous learning: Semantic search platforms leverage machine learning algorithms to continuously improve their understanding of user queries and preferences over time. By analyzing user interactions and feedback, these platforms adapt and refine their search capabilities, ensuring that search results become increasingly accurate and relevant over time.
Enhanced user experience: Ultimately, the goal of semantic search in enterprise settings is to enhance the overall user experience by making it easier and more intuitive for employees to access the information they need. By providing personalized search results that align with user preferences and context, semantic search platforms empower employees to extract actionable insights from complex datasets with ease, ultimately driving productivity and innovation across the organization.
Semantic vs. lexical or keyword search:
Semantic search represents a paradigm shift from traditional lexical or keyword-based searches, offering a more sophisticated approach to information retrieval. Let's explore how semantic search bridges the gap and overcomes the limitations of its predecessors:
Ambiguity resolution: One of the primary challenges with lexical searches is dealing with ambiguous queries. Words can have multiple meanings depending on context, leading to confusion in search results. Semantic search addresses this issue by considering the broader context of the query, enabling it to disambiguate and deliver more accurate results.
Synonym recognition: Lexical searches often falter when confronted with synonyms or related terms. For instance, a search for "car" might not retrieve results for "automobile." Semantic search, however, recognizes the semantic relationships between words and understands that these terms are interchangeable in certain contexts, ensuring that relevant information is not overlooked.
Language variations: Languages are dynamic, with variations in dialects, slang, and regional differences. Lexical searches struggle to accommodate these variations, leading to mismatches in search results. Semantic search, with its ability to grasp the underlying meaning of language, can interpret these variations and retrieve relevant information regardless of linguistic nuances.
Contextual understanding: Understanding the context of a query is crucial for delivering accurate search results. Lexical searches often treat queries in isolation, lacking the ability to consider the broader context in which the query is made. Semantic search, however, analyzes the context surrounding the query, taking into account factors such as user intent, previous interactions, and situational relevance to provide more contextually relevant outcomes.
Precision and recall: While lexical searches may excel in precision (retrieving exactly what was queried), they often fall short in recall (retrieving all relevant information). Semantic search strikes a balance between precision and recall by understanding the intent behind the query and retrieving information that may not contain the exact keywords but is conceptually related, thereby enhancing the overall search experience.
Examples of semantic search in workplace scenarios:

1. Project documentation retrieval: Suppose a project manager needs to gather information about past projects similar to the one they're currently undertaking. Instead of sifting through countless documents using traditional keyword searches, they can utilize semantic search.
By entering a query like "similar projects," the semantic search platform would understand the context and retrieve relevant documents based on project objectives, outcomes, team members, and other related factors, streamlining the research process significantly.
2. Employee skills matching: In a large organization, HR professionals often struggle to find the right candidates for specific roles or projects. Semantic search can facilitate this process by analyzing job descriptions, resumes, and employee profiles to identify relevant skills and experiences.
For instance, a recruiter looking for a data analyst with experience in Python and SQL could input a query like "data analysis skills," and the semantic search platform would match candidates based on their skills, certifications, and past projects, ensuring a more efficient and accurate talent search.
3. Customer support ticket resolution: Imagine a customer support agent tasked with resolving a complex issue raised by a customer. Traditionally, the agent would need to manually search through a vast repository of support documents, knowledge bases, and past ticket resolutions to find relevant information. However, with semantic search capabilities, the process becomes much more efficient.
Suppose a customer submits a ticket regarding a technical issue with a product. The support agent can input a query like "product troubleshooting" into the semantic search platform. Instead of relying solely on keyword matches, the platform understands the context of the query and retrieves relevant troubleshooting guides, FAQs, and solutions based on the specific product and issue.
Introducing Glean:
Glean is an enterprise search platform designed to enhance workplace productivity through intelligent information retrieval. By incorporating semantic search functionality with advanced machine learning algorithms, Glean goes beyond keyword matching to understand user intent, behavior, and preferences.
Glean seamlessly integrates with existing business applications, allowing employees to search across all organizational data repositories effortlessly. Whether it's customer records, project documents, or internal communications, Glean's intuitive interface and robust semantic search capabilities ensure that users find the precise information they need, precisely when they need it.
{{richtext-cta-component}}
In conclusion, semantic search represents a transformative leap forward in information retrieval, particularly within enterprise settings. By deciphering the intricacies of human language and context, semantic search engines empower employees to unlock the full potential of their organization's data, driving innovation, efficiency, and informed decision-making. With solutions like Glean leading the way, the future of workplace search no longer needs to be difficult.
Related articles
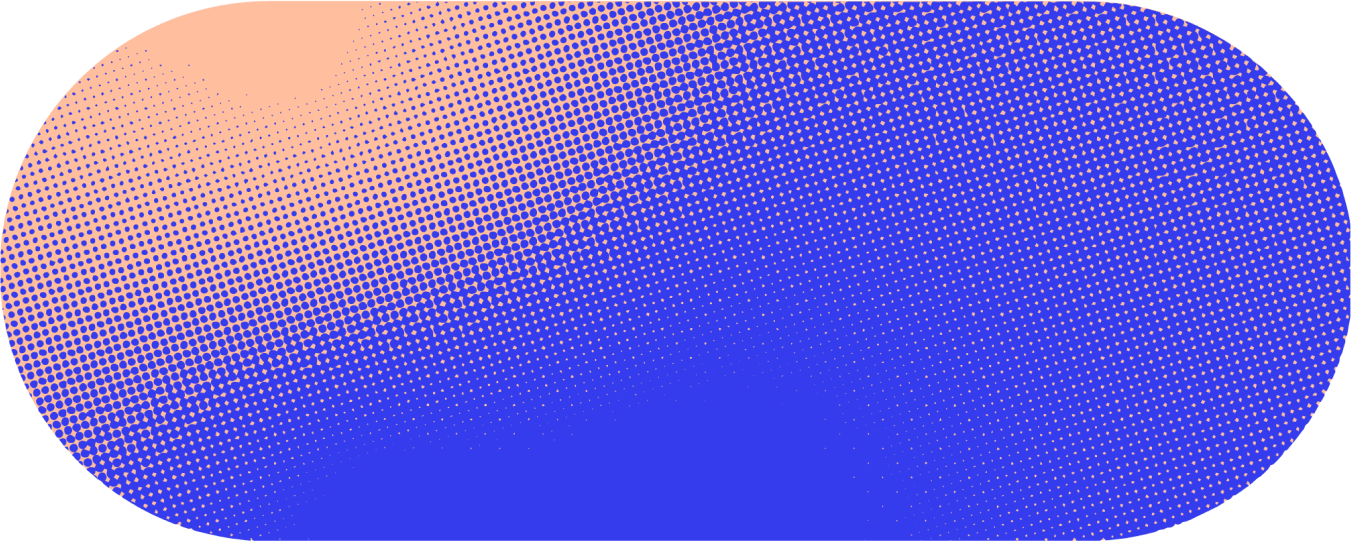